Effective Causal Analysis: Methods for Structure Learning and Explanations
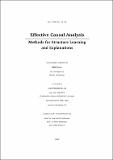
Auteur, co-auteurs
Type de référence
Date
2010Langue de la référence
AnglaisEntité(s) de recherche
ETH Zurich
Résumé
This study is concerned with causal analysis, an approach to multivariate-data analysis that aims to identify the structure of the data-generating process to understand how external interventions influence the system. In particular, causal knowledge can help understand the data better by preventing erroneous interpretations (e.g., Simpson's paradox), providing causal diagnostics to explain observed values, and evaluating alternative scenarios and policies that affect the data distribution. We study two key complementary aspects of causal analysis in depth: causal-structure learning and explanatory causal reasoning. The former deals with the computationally hard task of building a causal model; the latter uses the causal model to extract targeted contextual causal information for analysts. Our contributions to the structure-learning task are novel algorithms which improve upon current state of the art in accuracy and time complexity. We deal with the two main causal contexts: when all potential confounders are observed and when hidden confounders may exist. We argue that the presented algorithms, thanks to their reduced complexity, can tackle a new range of large causal problems. We then examine the causal-diagnostics task and present a new approach to evidence explanation: causal-explanation trees. Given some observed variables and a fully specified causal model, we show how to extract qualitative and quantitative information about how and why the system ended up in such a state. In addition to continuous empirical evaluation of the presented algorithms on standard benchmarks, we present in a final chapter two real-world applications of (subsets of) the introduced approaches. We discuss how validation of causal methods is difficult without artificial data, and show pragmatic alternatives to causal-model specification in adverse conditions.Ville d’édition
ZurichPays d'édition
SuisseDirecteur(s) de thèse
Buhmann, JoachimIntitulé du diplôme lié à la thèse
Ph.D. ThesisInstitution(s) liée(s) à la thèse
ETHZ & IBM ResearchURL permanente ORFEE
http://hdl.handle.net/20.500.12162/362Document(s) associé(s) à la référence
- Tout ORFEE
- Détail référence